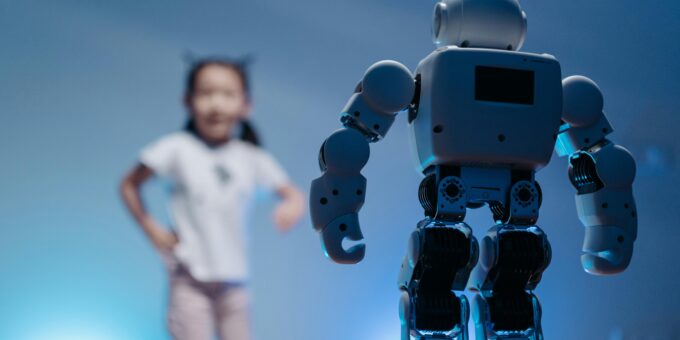
Machine learning (ML) is rapidly transforming industries across the globe, and insurance is no exception. In 2025, modern insurance solutions are heavily reliant on machine learning algorithms to streamline operations, assess risks, personalize customer experiences, and detect fraudulent activities.
This evolution marks a significant shift from traditional, manual insurance practices to data-driven, automated systems that improve efficiency and accuracy. With its ability to analyze large datasets, identify patterns, and make predictions, machine learning has become the backbone of innovation in the insurance sector.
In this article, we will explore how machine learning is reshaping insurance solutions, diving into key applications, challenges, and its future potential.
Introduction
The insurance industry is undergoing a digital transformation, driven by the increasing adoption of machine learning. Historically, insurers faced challenges such as inefficient claims processing, difficulty in fraud detection, and limited personalization in policy offerings. These bottlenecks not only increased operational costs but also led to poor customer experiences.
Machine learning is addressing these pain points by automating tasks, providing predictive insights, and enabling smarter decision-making. From understanding customer behaviors to optimizing policy pricing, the role of ML in modern insurance is pivotal.
But why has machine learning become such a critical component of insurance solutions? The answer lies in its ability to process and analyze vast amounts of structured and unstructured data. Unlike traditional systems, ML algorithms continuously learn and adapt, enabling insurers to stay ahead in an increasingly competitive market.
Machine Learning in Insurance: Core Applications
Machine learning is revolutionizing the insurance industry through its diverse applications. Let’s explore how ML is being applied to key areas of modern insurance solutions.
1. Claims Processing Automation
Claims processing has historically been a time-consuming and resource-intensive task for insurers. Machine learning has introduced automation, significantly speeding up this process while reducing errors.
- Document Analysis with NLP: Natural Language Processing (NLP) algorithms extract relevant information from claim documents, eliminating the need for manual reviews.
- Image Recognition for Damage Assessment: ML-powered image recognition tools analyze photos of damaged vehicles, properties, or other insured items to provide instant repair estimates.
- Automated Decision-Making: Machine learning models analyze claims data to determine whether a claim should be approved, denied, or flagged for further investigation.
For example, insurers like Allstate use machine learning to analyze crash photos and provide repair cost estimates within minutes, enhancing customer satisfaction.
2. Risk Assessment and Underwriting
Underwriting is the foundation of the insurance industry, and machine learning is making it faster, more accurate, and more personalized. Traditional underwriting relied on historical data and static models, whereas ML enables dynamic and data-driven risk evaluation.
- Predictive Analytics: Machine learning predicts the likelihood of claims or accidents by analyzing large datasets, including driving records, health data, and lifestyle habits.
- Dynamic Pricing Models: ML adjusts premiums in real-time based on changing risk factors, such as driving behavior recorded by telematics devices or wearable health trackers.
- Customer Segmentation: Machine learning clusters customers into risk groups based on their behavior, demographics, and historical data, helping insurers offer more tailored policies.
For instance, health insurers are leveraging wearable devices to collect continuous health data, which ML algorithms analyze to create personalized health insurance premiums.
3. Fraud Detection and Prevention
Fraudulent claims have long been a challenge for insurers, costing billions annually. Machine learning is transforming fraud detection by identifying anomalies and suspicious patterns in claims data.
- Anomaly Detection: ML algorithms identify deviations from normal patterns, such as unusual claim amounts or inconsistencies in policyholder data.
- Behavioral Analytics: By analyzing customer behavior, machine learning detects signs of fraud, such as repetitive claims or fabricated incidents.
- Voice Analysis: NLP-powered tools analyze voice patterns in recorded claims interviews, identifying signs of deception.
For example, Zurich Insurance uses machine learning to detect fraud by analyzing millions of claims and flagging those with unusual patterns for further review.
4. Personalized Customer Experiences
In the era of personalization, customers expect insurance solutions tailored to their unique needs. Machine learning enables insurers to analyze customer data and provide highly personalized services.
- Policy Recommendations: ML models recommend insurance products based on a customer’s life stage, habits, and preferences.
- Dynamic Engagement: Machine learning predicts when customers are likely to need additional coverage, such as after a major life event like a marriage or home purchase.
- Chatbots and Virtual Assistants: AI-driven chatbots use machine learning to provide 24/7 support, answering questions about policies, claims, and payments.
Insurers like Progressive are already using ML-powered tools to offer usage-based insurance (UBI) policies, which track individual driving habits and reward safe drivers with lower premiums.
5. Predictive Analytics for Risk Management
Predictive analytics, powered by machine learning, is helping insurers adopt a proactive approach to risk management. Instead of reacting to events, insurers can now predict and mitigate risks before they occur.
- Catastrophe Modeling: ML analyzes historical data to predict natural disasters like hurricanes, earthquakes, or floods, enabling insurers to prepare for claims surges.
- Cyber Risk Assessment: With the rise of cyber threats, machine learning evaluates vulnerabilities in digital ecosystems and recommends tailored cyber insurance solutions.
- Business Risk Monitoring: ML tracks market trends, economic shifts, and global disruptions, helping insurers identify potential risks to their portfolios.
These predictive capabilities position insurers as proactive partners, rather than reactive service providers.
6. Telematics and IoT Integration
The integration of machine learning with the Internet of Things (IoT) is creating smarter, data-driven insurance solutions.
- Auto Insurance: Telematics devices in vehicles record driving behaviors such as speed, braking, and mileage. ML analyzes this data to calculate premiums based on real-time driving habits.
- Home Insurance: Smart home devices like water leak detectors and smoke alarms generate data that ML systems analyze to offer discounts for proactive risk management.
- Health Insurance: Wearable devices track fitness metrics, which ML uses to customize health insurance policies and offer rewards for healthy behaviors.
This integration of IoT and ML provides insurers with unprecedented levels of insight into customer behavior and risk.
Challenges in Implementing Machine Learning in Insurance
While machine learning offers immense potential, its implementation comes with challenges that insurers must address:
- Data Quality: Poor or incomplete data can result in inaccurate predictions and decisions.
- Bias in Algorithms: Machine learning models can inherit biases from training data, leading to unfair pricing or discrimination.
- Regulatory Compliance: Insurers must ensure that ML-driven processes comply with data protection laws like GDPR and CCPA.
- Ethical Considerations: Transparency in ML decisions is critical to maintain trust with customers and regulators.
Addressing these challenges will be key to unlocking the full potential of machine learning in insurance.
FAQs
What is the role of machine learning in insurance?
Machine learning automates processes, predicts risks, detects fraud, and personalizes customer experiences, making insurance solutions more efficient and data-driven.
How does machine learning improve claims processing?
ML automates claims filing, uses image recognition for damage assessment, and processes claims data to provide faster resolutions.
Can machine learning detect insurance fraud?
Yes, ML detects anomalies, analyzes behavioral patterns, and uses tools like voice analysis to identify fraudulent claims in real time.
What is predictive analytics in insurance?
Predictive analytics uses machine learning to forecast risks, such as claims likelihood or natural disasters, helping insurers proactively manage policies.
How does machine learning personalize insurance policies?
ML analyzes customer data, such as demographics and behaviors, to recommend tailored policies and adjust premiums dynamically.
What are the challenges of implementing machine learning in insurance?
Challenges include ensuring data quality, addressing algorithmic bias, complying with regulations, and maintaining transparency in AI-driven decisions.
Machine learning is revolutionizing the insurance industry by automating processes, enhancing risk assessment, detecting fraud, and delivering personalized experiences. As insurers embrace ML, they are not only improving operational efficiency but also redefining how they interact with customers.
While challenges like data quality and regulatory compliance remain, the benefits of machine learning far outweigh the risks. By addressing these hurdles, insurers can unlock the full potential of ML and lead the way in providing smarter, faster, and more customer-centric insurance solutions.
As we look ahead, it’s clear that machine learning is not just a tool—it’s the cornerstone of modern insurance solutions.