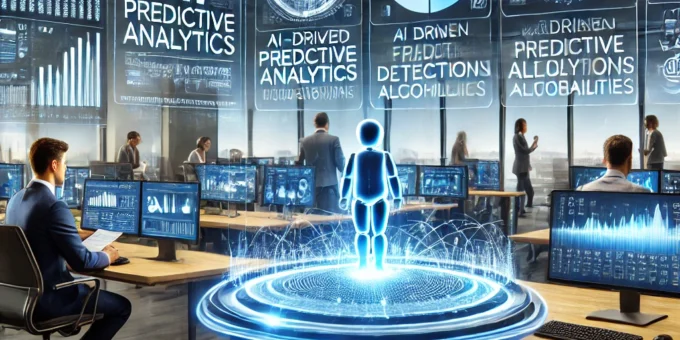
How to Leverage AI for Smarter Insurance Risk Management
In the ever-evolving world of insurance, managing risk effectively is at the heart of every decision. Traditional methods of assessing and managing risk are increasingly giving way to artificial intelligence (AI), which has the power to analyze vast datasets, predict trends, and enhance decision-making. With AI, insurers can streamline risk assessment, reduce losses, and offer more personalized policies to customers.
This guide explores how to leverage AI for smarter insurance risk management and provides actionable insights to implement this technology successfully.
Introduction
Insurance risk management involves identifying, evaluating, and mitigating potential risks that could lead to financial losses for insurers. Traditionally, this process has relied on historical data, manual calculations, and expert judgment. However, as the volume and complexity of data have grown, these methods are no longer sufficient.
AI technologies such as machine learning (ML), predictive analytics, and natural language processing (NLP) enable insurers to process vast amounts of data quickly and accurately. By uncovering patterns and insights, AI helps insurers predict risks, detect fraud, and optimize policies, leading to smarter and more efficient risk management practices.
The Role of AI in Insurance Risk Management
AI enhances every aspect of insurance risk management, from underwriting to claims processing. Here’s how:
- Data Processing: AI analyzes massive datasets in real-time, providing insights that human analysts might miss.
- Predictive Modeling: Algorithms forecast potential risks based on historical and real-time data.
- Automation: Repetitive tasks, such as risk scoring, are automated, saving time and resources.
- Fraud Detection: AI identifies anomalies and suspicious behavior more effectively than traditional methods.
Key Applications of AI in Insurance Risk Management
1. Advanced Risk Assessment
AI improves the accuracy of risk assessment by integrating data from various sources, including:
- Historical Claims Data: Identifies patterns and trends.
- IoT Devices: Monitors real-time data, such as driving habits or home security.
- Weather Models: Predicts natural disasters and their potential impact.
- Example:
An insurer might use AI to evaluate risk for a homeowner’s policy by analyzing local weather data, crime rates, and the condition of the property.
2. Predictive Analytics for Proactive Decision-Making
Predictive analytics allows insurers to foresee potential risks before they occur.
- How It Works:
- Analyzes customer behavior, industry trends, and environmental factors.
- Uses machine learning models to forecast future events, such as increased claims in high-risk areas.
- Example:
A health insurer could predict which customers are at higher risk of developing chronic conditions based on wearable device data and medical history, enabling proactive interventions.
3. Fraud Detection and Prevention
Fraudulent claims are a significant challenge in the insurance industry, costing billions annually. AI strengthens fraud detection by:
- Identifying Patterns: Machine learning algorithms detect irregularities in claims data.
- Behavioral Analysis: NLP tools analyze customer interactions for inconsistencies.
- Real-Time Alerts: Flags potential fraud as it occurs, allowing for immediate action.
- Example:
Zurich Insurance uses AI to detect fraud by analyzing historical claims data and identifying anomalies in patterns, such as duplicate claims or exaggerated losses.
4. Catastrophe Modeling
AI improves catastrophe modeling by predicting the frequency and severity of natural disasters.
- Key Features:
- Analyzes historical weather data, satellite imagery, and climate models.
- Simulates disaster scenarios to estimate potential losses.
- Example:
AI helps property insurers assess the financial impact of hurricanes, earthquakes, or floods, enabling better preparedness and accurate pricing.
5. Dynamic Pricing and Risk-Based Premiums
AI enables insurers to adjust premiums dynamically based on real-time risk factors.
- Telematics Data: Tracks driving behavior to determine auto insurance rates.
- Health Monitoring: Uses wearable devices to offer personalized health premiums.
- Climate Risk: Adjusts property insurance rates based on weather patterns.
- Example:
Progressive’s Snapshot program uses AI to monitor driving habits, offering discounts to safe drivers while charging higher premiums for risky behavior.
6. Portfolio Risk Management
AI helps insurers manage risk across their entire portfolio by analyzing aggregate data.
- Benefits:
- Identifies overexposure in specific risk categories.
- Balances risks across diverse policy types.
- Example:
AI could reveal that an insurer is overexposed to flood-prone areas, prompting diversification of its portfolio.
Benefits of Using AI for Risk Management in Insurance
1. Improved Accuracy
AI eliminates human error and provides precise risk assessments, leading to better decision-making.
2. Faster Decision-Making
Real-time data analysis accelerates processes such as underwriting, claims approval, and fraud detection.
3. Cost Savings
By automating risk assessment and detecting fraud, AI reduces administrative costs and minimizes financial losses.
4. Enhanced Customer Experience
AI enables personalized policies, proactive risk mitigation, and faster claims processing, improving customer satisfaction.
5. Competitive Advantage
Early adopters of AI gain an edge by offering innovative solutions and efficient services.
Challenges of Implementing AI in Risk Management
1. Data Privacy Concerns
Handling sensitive customer information requires strict compliance with data protection laws like GDPR or CCPA.
- Solution:
Implement robust encryption and anonymization techniques to secure data.
2. Integration with Legacy Systems
Integrating AI tools with outdated infrastructure can be complex and costly.
- Solution:
Adopt modular AI solutions that can work alongside existing systems without requiring a complete overhaul.
3. Algorithm Bias
AI models can unintentionally reinforce biases present in the training data, leading to unfair decisions.
- Solution:
Regularly audit algorithms for bias and ensure diverse datasets during model training.
Steps to Implement AI for Smarter Risk Management
1. Identify Key Risk Areas
Focus on areas where AI can provide the most value, such as fraud detection, underwriting, or catastrophe modeling.
2. Partner with Technology Providers
Collaborate with AI vendors or InsurTech firms to access specialized tools and expertise.
3. Build Data Infrastructure
Invest in robust data collection, storage, and processing capabilities to ensure high-quality input for AI models.
4. Train Your Team
Educate employees on AI applications and provide training to use these tools effectively.
5. Start with Pilot Projects
Test AI solutions on a smaller scale to measure their impact and address any challenges before full-scale implementation.
Real-World Examples of AI in Insurance Risk Management
1. AXA
Uses AI to assess climate-related risks, helping customers mitigate the impact of natural disasters.
2. Liberty Mutual
Leverages machine learning to evaluate underwriting risks and streamline policy approvals.
3. State Farm
Deploys AI to analyze driving data from connected cars, offering tailored auto insurance policies.
FAQs
How does AI improve risk management in insurance?
AI enhances risk management by analyzing data more accurately, predicting future risks, detecting fraud, and enabling dynamic pricing.
Can AI help reduce insurance premiums?
Yes, AI rewards low-risk customers with personalized discounts based on data such as driving habits or health metrics.
What are the challenges of using AI in insurance?
Challenges include data privacy concerns, integration with legacy systems, algorithm bias, and high implementation costs.
Which AI technologies are most commonly used in insurance?
Machine learning, predictive analytics, natural language processing, and image recognition are the most common AI technologies in insurance.
Is AI suitable for small insurance companies?
Yes, scalable AI solutions, such as cloud-based platforms, allow small insurers to benefit from AI without significant upfront investment.
What is the future of AI in insurance risk management?
The future includes greater use of real-time data, more accurate predictive models, and deeper integration of AI into all aspects of risk assessment and claims processing.
AI is revolutionizing insurance risk management by providing more accurate assessments, faster decision-making, and personalized customer experiences. By leveraging AI, insurers can predict risks, detect fraud, and optimize pricing strategies, ultimately improving profitability and customer satisfaction.
While challenges such as data privacy and integration exist, adopting AI thoughtfully and strategically will enable insurers to stay ahead in an increasingly competitive market. As AI continues to evolve, it will remain a cornerstone of smarter and more efficient insurance risk management.